Upcoming Events!
Past Events
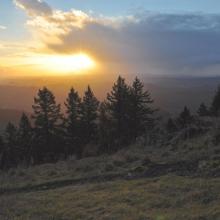
Abstract: Understanding how the brain gives rise to behavior is a central question in neuroscience...
Abstract: Understanding how the brain gives rise to behavior is a central question in neuroscience, but this endeavor is hampered by the difficulty of quantitatively measuring and modeling complex behavior. This problem is especially pronounced in studies of social behavior using animal models which often employ freely-moving and naturalistic behavioral paradigms. In this talk, I will highlight some of our recent work in building computational tools driven by deep learning and computer vision for robust motion capture of socially-interacting animals, including flies, bees, mice, marmosets and humans. The postural dynamics captured by these technologies enable highly quantitative behavioral analyses at unprecedented resolution; towards the end of the talk I will outline the efforts we're currently undertaking to use these types of data to model the brain, as well as how we've been applying these tools to other domains such as plant biology. <a href="https://urldefense.com/v3/__https://talmolab.org/__;!!C5qS4YX3!UFiWhPHO… more</a>|full_html
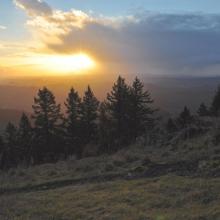
<p>I am an Evolutionary Immunologist at the Biology Department, University of New Mexico. I love the...</p>
<p>I am an Evolutionary Immunologist at the Biology Department, University of New Mexico. I love the complexity of immune systems, from bacteria to humans. My research focuses on mucosal immunity and its evolution in vertebrates. Mucosal surfaces are at the interface between hosts and the environment and therefore integrate the external and internal signals.</p>
<p>My laboratory has very diverse research interests and we currently have active projects in trout, zebrafish, lungfish, mice and humans. These projects reflect the diversity and breath of the research that takes place at the UNM Biology Department.</p>
<p>In the recent years, we have specialized in the understanding of nasal immunity and the neuroimmune interactions that occur in the olfactory-central nervous system axis in response to microorganisms.</p>
|full_html
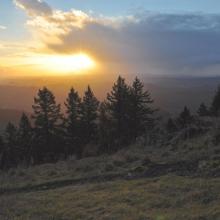
Transcriptomics has revealed the exquisite diversity of cortical inhibitory neurons, but it is not...
Transcriptomics has revealed the exquisite diversity of cortical inhibitory neurons, but it is not known whether these fine molecular subtypes have correspondingly diverse activity patterns in the living brain. Here, we show that inhibitory subtypes in primary visual cortex (V1) have diverse correlates with brain state, but that this diversity is organized by a single factor: position along their main axis of transcriptomic variation. We combined in vivo 2-photon calcium imaging of mouse V1 with a novel transcriptomic method to identify mRNAs for 72 selected genes in ex vivo slices. We used transcriptomic clusters (t-types) to classify inhibitory neurons imaged in layers 1-3 using a three-level hierarchy of 5 Families, 11 Classes, and 35 t-types. Visual responses differed significantly only across Families, but modulation by brain state differed at all three hierarchical levels. Nevertheless, this diversity could be predicted from the first transcriptomic principal component, which predicted a cell type’s brain state modulation and correlations with simultaneously recorded cells. Inhibitory t-types with narrower spikes, lower input resistance, weaker adaptation, and less axon in layer 1 as determined in vitro, fired more in resting, oscillatory brain states. Types with the opposite properties fired more during arousal. The former cells had more inhibitory cholinergic receptors, and the latter more excitatory receptors. Thus, despite the diversity of V1 inhibitory neurons, a simple principle determines how their joint activity shapes state-dependent cortical processing.
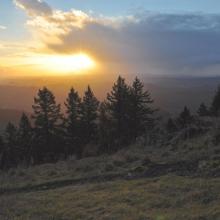
Recent advances in machine learning have shown that deep neural networks (DNNs) can provide powerful...
Recent advances in machine learning have shown that deep neural networks (DNNs) can provide powerful and flexible models of neural sensory processing. In the auditory system, standard linear-nonlinear (LN) models and their derivatives are unable to account for high-order cortical representations, and DNNs may provide additional insight cortical sound processing. Deep learning can be difficult to implement with relatively small datasets, such as those available from single neuron recordings. To address this limitation, we developed a population encoding model, a network model that simultaneously predicts activity of many neurons recorded during presentation of a large, fixed set of natural sounds. This approach defines a spectro-temporal space that is shared by all the neurons and pools statistical power across the population. We tested a range of DNN architectures on data from primary and non-primary auditory cortex. DNNs performed substantially better than LN models. Moreover, the DNNs were highly generalizable. The output layer of a model pre-fit using one population of neurons could be fit to different single units and/or different stimuli, with performance close to that of neurons in the original model. These results indicate that population encoding models capture a general set of computations performed by auditory cortex and that the model itself can be analyzed for a general characterization of auditory cortical representation. |full_html